Reducing Costs of AI-based Heart Monitors
Real-Time Detection & Prediction of Heart Diseases via ECG Data Using Neural Networks and Distributed Computing.
Real-Time Detection & Prediction of Heart Diseases via ECG Data Using Neural Networks and Distributed Computing.
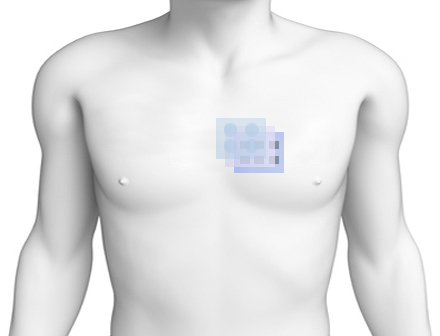
The Motivation
Conventional systems that detect and predict heart diseases through ECG data could be vaguely classified into two types: those that provide real-time detection with high accuracy and performance but are large and expensive, and those that are portable or wearable but have limited real-time functionality with low accuracy and lacks crucial features. Our work focused on bringing together the best of both without compromising on affordability.
Developments
We first developed an AI model that would detect and predict heart diseases from real-time data. We then deployed our model over a Fog-inspired network that allows us to run lightweight computations locally on the user-end wearable device, while the distributed servers would handle all the heavy lifting. The server network would then incrementally train the Neural Network to regularly update its parameters, which would periodically be sent over to the user-end Edge Devices. Since the Edge Devices would need to only collect the ECG data and use the trained model to predict and detect abnormalities, it can be designed to meet much-needed affordability standards.
427
79
180
My Role
My responsibilities included optimisation of AI architecture, EDGE Computing System, Embedded System design, etc.
The Team
The team consisted of three student members from NITW and one faculty member, Mr SKLV Sai Prakash.
Status
The technical details of our work have now been published by Springer, and can be found here.
My Role
My responsibilities included optimisation of AI architecture, EDGE Computing System, Embedded System design, etc.
The Team
The team consisted of three student members from NITW and one faculty member, Mr SKLV Sai Prakash.
Status
The technical details of our work have now been published by Springer, and can be found here.